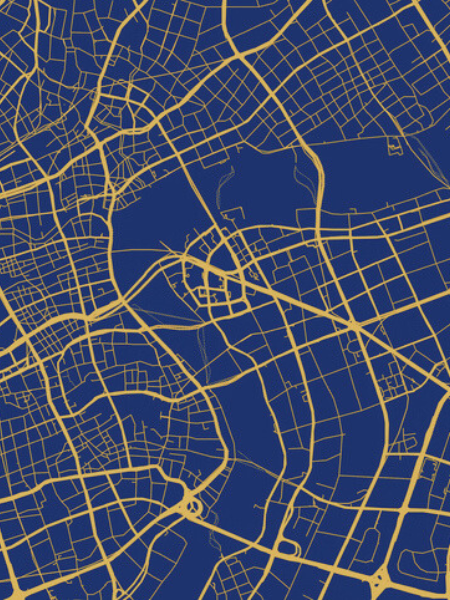
The use of artificial intelligence (AI) is not new for UK financial services firms. It is already modernising the banking, insurance and payments sectors, who use it to enhance customer service, personalise insurance cover and detect suspicious payment transactions. The regulators themselves are also hopping on the bandwagon – the UK's Financial Conduct Authority (FCA) announced last year that it is using AI-based models to help tackle fraud. But despite some tangible benefits, the financial services industry is still exercising caution when it comes to AI.
Last November, UK Finance (in partnership with Oliver Wyman) published a report on the state of play of AI adoption, which looked at both benefits and risks, and its impact on UK financial services (Report). The Report is based on a survey that UK Finance conducted among 23 of its members, representing a cross-section of the UK financial services sector. In this article, written for Compliance Monitor, Lucy Hadrill and Katie Simmonds of Womble Bond Dickinson discuss the Report and consider whether AI has the potential to change the game for financial services.
How is AI used in financial services?
The Report found that the majority of UK financial institutions view AI in a positive light, appreciating the opportunities that it presents to drive efficiencies and improve customer experiences. In fact, over 90% of respondents to the UK Finance survey are already successfully using AI in their businesses and are reaping the rewards.
The deployment seen so far is in areas such as fraud detection, KYC and in back office functions. Banks, for example, which to date have had to rely on traditional transaction monitoring systems, are adding enhanced AI components to their existing systems, which is enabling them to detect transactional patterns and anomalies which previously flew under the radar. With the alarming uptick in fraud and other financial crimes, AI is helping banks to be preventative rather than reactive in their approach. Lenders are also using AI to determine a borrower's creditworthiness by using data to assess the likelihood of a default.
In insurance, AI can be used to monitor and analyse costs, claims etc. to help predict risk and enable insurers to price more accurately. It is also being used to analyse customer behaviour and predict customer intent which insurers can harness to better personalise the customer experience.
But it's not just firms. The regulators themselves are also making use of emerging tech. In a recent speech, the FCA announced that it is using AI-based models to help tackle fraud and that its Advanced Analytics unit is making use of new supervisory technology (suptech) to help protect consumers and markets. For instance, it has developed web-scraping and social media monitoring tools which can detect, review and triage potential scam websites.
These are all examples of predictive AI, that is, models which analyse data to identify patterns in past events, anticipate behaviours and make predictions about future trends. But what about generative AI? What is it? What benefits can it bring?
What about generative AI?
Generative AI (such as ChatGPT) can be used to generate new content, text, images, audio, video or code. Whilst in its relative infancy, the potential of generative AI is gaining attention within the financial services sector, with organisations considering how best to integrate it alongside their existing predictive AI models.
The survey found that over 75% of financial institutions expect the same, if not higher, benefits from generative AI compared to predictive AI, and more than 70% of use cases are in already in the proof of concept or pilot stage. However, we are not likely to see the results of this early adoption for maybe up to five years, depending on the quality of the relevant data and how easily the new technology integrates into existing systems.
The best results will probably come from firms using predictive and generative AI together as each AI system has its own strengths. For instance, generative AI could be used alongside predictive AI for anomaly detection purposes or firms might use it to assess the performance of their existing predictive models by creating reports and summaries of their analyses. It could also generate synthetic data to help better train the existing predictive models. Embedding complementary systems that differ but reinforce each other will take time but the Report encourages adopting an holistic approach to AI, enabling businesses to identify and enable such synergies.
Although it will take time to do so, generative AI could transform certain areas of business operations in a way which predictive AI cannot, in particular in process automation and customer service functions – e.g. generative AI can assist with translating code between languages, document search functions and generating marketing content.
The Report includes two case studies of how regulated firms have already deployed generative AI:
- In September 2023, Marsh McLennan launched its assistant tool, LenAI, which uses ChatGPT as its underlying model but maintains the security of the firm's data within its cloud environment. Within 30 days of the launch, 15,000 people were using LenAI to summarise documents, assist with coding and to supplement brainstorming processes.
- In 2021, HSBC and Google Cloud launched an anti-money laundering dynamic risk assessment system which analyses transaction patterns and KYC information to generate risk scores for groups of retail and commercial customers. This tool allows HSBC to more easily identify financial crime and streamline the resultant investigation workflow.
So, whilst firms are currently very much experimenting with generative AI, the potential benefits it can offer are clear. 73% of those survey respondents in the pilot stage are already identifying significant cost and efficiency use cases. But as well as cutting costs and driving efficiency, generative AI has the potential to generate revenue through product personalisation and importantly to improve the customer experience, thereby helping firms to maintain a competitive edge.
Implementing generative AI
What do financial services firms need to be aware of when considering whether to implement generative AI?
Generative AI models have key capabilities, such as content generation, that make them preferable to predictive AI for certain tasks. But they also have their limitations. For instance, the Report found that generative AI tools lack a grounded base knowledge and are unaware of what they know and don't know. They also tend to "hallucinate", that is, produce an output that is factually incorrect but which is presented so confidently that the human recipient believes it. Two New York lawyers were stung by this particular "quirk" of generative AI last summer – they were fined for submitting a legal brief which included fictitious case citations generated by ChatGPT. This emphasises the need for users of AI systems to interrogate and verify that any outputs of an AI system are correct. This is likely to be through a combination of human oversight and implementation of policies supporting how AI decisions are being made and relied upon.
It is also important to note that different data is required to train predictive and generative AI systems. Predictive models normally rely on the organisation's own data whereas generative models are trained using a wider data pool taken from various public and purchased sources. The costs involved in creating and training a base generative AI model means that financial institutions are unlikely to do this themselves. This presents data privacy challenges, which we will touch on later.
So, adoption of generative AI will not be without its challenges, and financial institutions will need to overcome barriers such as technical limitations and data quality. The Report sets out several key considerations that firms should take into account:
High build and deployment costs
- Are your existing systems ready to embed generative AI?
- Dataset maintenance – large datasets of potentially private data need to be built and maintained
- Model sourcing – which tool do you buy?
- Model training – getting the data and training the model takes time and money
- Tools and outputs need to be specialised for industry
- Misuse of tools could be a serious issue – how will you train employees on the new technologies?
Organisational changes
- As noted above, employees will need sufficient training to use the new tools effectively
- Integration at scale is tricky and time consuming
- Does the model solve the underlying problems/enhance existing systems or is it simply window dressing?
Data quality, privacy and security
- Poor data means skewed results and reduced accuracy – how will you ensure data quality?
- Data remediation tools are important to avoid bias
- Data management is key to maintain corporate security
- Monitoring model outputs is key
Whilst it is easy to see that AI can drive efficiency, it is tougher to prove how it enhances effectiveness, so firms also need to consider how they measure and manage tangible success. For example, in the context of financial crime, should success be measured against the ratio of system alerts to suspicious activity reports? The challenge for industry is agreeing on what "effective" looks like. Firms can determine this by starting with areas where they already know and can recognise what effective looks like.
What are the risks?
As with any new technology, the use of generative AI is not without risk. And although firms have taken steps to mitigate risks that are common to both predictive and generative AI, the latter introduces a new layer of challenges.
One key concern is that generative AI may lead to poor customer outcomes which in turn would lead to difficulties in complying with the Consumer Duty and to potential reputational damage. For instance, problems with system design or training data can lead to discriminatory or unfairly biased content. As noted earlier, generative AI models can also be unpredictable and tend to "hallucinate". Such hallucinations can lead to negative customer outcomes in fraud detection, for example, if the model generates false positives based on its assumptions about what forms fraudulent behaviour. These models can also be misused and manipulated by users which has the potential to wreak havoc and cause reputational damage, particularly in customer service functions. This happened recently in a different sector where a customer was able to make a parcel delivery company's chatbot swear and write a poem criticising the delivery company's customer services.
Another major area of concern is around data security, privacy and intellectual property breaches. The Report explains that copyright and IP infringements are likely with generative models, particularly where copyrighted text or media have been used as training data, as this can lead to tainted outputs containing protected extracts. Data breaches are also a significant risk – personal information can be extracted from a model's training data simply by asking the model to provide it. Private information can also be extracted from the model by malicious users. From a cybersecurity perspective, we always recommend asking – what would happen if this data got hacked? Vast amounts of data is processed by AI systems, which presents heightened risks, particularly in relation to data exfiltration, data manipulation and data poisoning risks. It is therefore essential to protect the underlying data with extensive controls.
Financial institutions can mitigate these risks by adapting their existing risk frameworks to account for generative AI (or AI more widely) and by educating their businesses on the risks and correct usage of AI. It is also fundamental to keep an element of human oversight of any AI tools. This could include implementing an approval process for critical outputs, such as marketing materials, or monitoring performance metrics. Firms should also consider operational mitigations, for instance building in certain software solutions to prevent the model from producing content that references sensitive or inappropriate topics.
Regulatory landscape
It is also important to consider the policy landscape. Indeed, the Report found that 65% of survey respondents are concerned about the direction of travel of regulation when thinking about whether to adopt AI. This is despite the UK's flexible regulatory approach being technology-neutral.
So where have we got to on regulation? The government's AI Regulation white paper consultation set out a provisional principles-based approach to legislation which it intended would align with existing regulatory regimes. In its response to the consultation, the government confirmed this agile approach and announced its plans for implementation, including spending £10 million to prepare and upskill regulators to address the risks and harness the benefits of AI. A separate c.£90 million fund will help launch nine AI research hubs across the UK and will enable the regulators to develop their own research and practical tools to monitor AI adoption in their sectors, e.g. new technical tools for examining AI systems.
Hopefully this announcement will spur the UK regulators into action. To date, they have been dragging their heels somewhat, with consultation ongoing. This is in stark contrast to developments in the United States, Singapore and in the EU. The FCA and the Bank of England published a discussion paper in October 2022 seeking input on the safe and responsible adoption of AI and the role of policy and regulation. The feedback statement showed that industry respondents generally support a principles-based, outcomes-focussed approach to AI regulation, as this would allow for flexibility as the AI landscape, and generative AI in particular, evolves.
But the currently unclear regulatory expectations on AI are a challenge and there are numerous policy considerations for further discussion. The first is whether AI should be defined in law. A definition could clarify legal compliance for firms, but there is a risk that any definition could quickly become outdated or misaligned with AI systems in practice. A more flexible alternative would be guidance from the regulators or government but this could lead to differences between the financial and other sectors which may complicate compliance for those firms operating in multiple sectors.
Another concern for firms operating cross-sector is whether expectations would differ and conflict among regulators. The survey respondents noted that the application of the AI fairness principle was likely to cause future tension as regulatory priorities relating to fairness in existing regimes (FCA rules, GDPR etc.) may change and conflict over time. One way to address this could be to nominate a single authority to be responsible for all AI regulation but this approach may not be effective in practice. Instead, the government Whitepaper suggested creating a 'central function' to coordinate different regulators and manage multi-sector issues. In a similar vein, firms operating cross-border will need to be able to comply with AI regulations in multiple jurisdictions. The Report notes that the extraterritorial reach of the EU AI Act (which is the most advanced AI law globally) is particularly relevant to UK firms. Adopting equivalent legislation in the UK would allow firms operating in both the UK and EU to more easily comply with AI regulation. However, this approach would mean forfeiting the benefits of a more flexible and sector-driven regime.
These are just a few examples but there is clearly lots to consider in relation to regulating AI. But whilst the regulatory picture remains unclear, firms need to be in a position to explain and communicate their use of AI to the regulators. They need to be confident of understanding what tools they have purchased or deployed, what those tools are doing and how the firm is testing them. Firms also need to be in a position to collaborate with regulators and explain any issues they have faced in their use of AI, e.g. bugs, biases and blind spots, but also how they protect data and confidential information about clients and business partners, and vulnerable customers.
Conclusion
The implementation of AI has undoubtedly marked a transformative phase in the UK financial services sector, with predictive AI already making significant strides in areas such as fraud detection and KYC. Generative AI also holds immense promise and is gaining attention for its potential to revolutionise process automation and customer service functions. But despite the evident benefits, the Report also highlights the risks and challenges of generative AI, as well as industry concerns about usage and data privacy.
On the regulatory front, the evolving policy landscape adds another layer of complexity. While the UK regulators continue to explore a principles-based approach, firms must be prepared to collaborate with the regulators, articulate their AI usage and address potential challenges in a rapidly changing regulatory environment.
What's clear is that it is now impossible to ignore the power AI has to transform the financial services arena and the clear benefits it can bring to the way in which financial products and services are delivered to customers. While challenges persist, there can be no doubt that AI has the potential to really change the game and cement the UK's position as the global leader in financial services.
This article was written for, and published in, Compliance Monitor.